Tie together an algorithm, an
exchange-traded fund and an academic study finding an anomaly in the markets,
and voilà! You have a formula for making money. Trouble is, it turns out that
most of the supposed anomalies academics have identified don’t exist, or are
too small to matter.
A new study making waves in
quantitative finance tested 447 anomalies identified by academics and found
more than eight out of 10 vanish when rigorous tests are applied. Among those
failing to reach statistical significance: one anomaly recently set out by the
godfathers of quantitative finance, Nobel-winning economist Eugene Fama and his
colleague Kenneth French.
The study, “Replicating
Anomalies,” published this week by Kewei Hou and Lu Zhang at Ohio
State University and Chen Xue at the University of Cincinnati, is the biggest
test of examples of inefficient markets carried out so far. The trio applied
consistent analysis to the supposed anomalies, used the same database of stocks
and set higher standards for statistical significance. Simply reducing the influence
of the plethora of rarely traded penny stocks—which make up just 3% of market
value but 60% of all listings—by using market capitalization weightings made
more than half of past findings no longer significant.
Messrs. Hou, Xue and Zhang warn
that academics have been fiddling the statistics to come up with interesting
findings, known to statisticians as data mining or p-hacking. “The anomalies
literature is infested with widespread p-hacking,” they write.
It isn’t all bad news for
investors and those trying to make a living flogging what have become known as
“factors.” The research confirmed that the most popular factors have indeed
outperformed the market over long periods even when faced with rigorous tests,
but found much smaller returns than previous studies estimated.
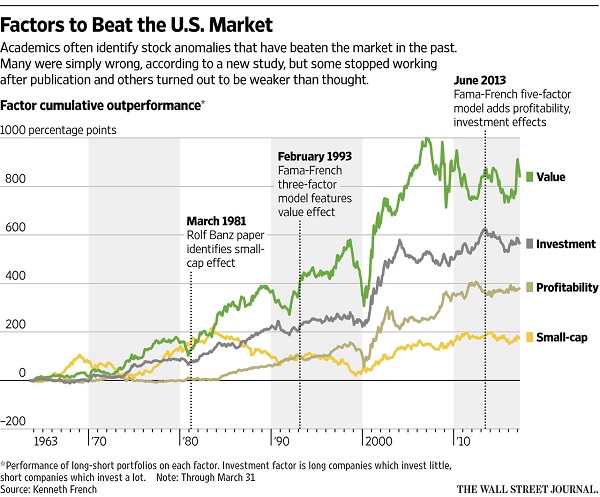
Market anomalies that passed the
new study’s tests included several of the biggest. Cheap stocks indeed beat
expensive ones; share prices have momentum; companies that invest a lot
underperform, and quality of earnings matters. Known as value, momentum,
investment and quality, these have become the biggest of the so-called “smart
beta” ETFs sucking in tens of billions of dollars.
A lot depends on exactly how the
factors are implemented, though, and the researchers dismissed one of the
industry-standard Fama-French factors as statistically insignificant: Companies
with high operating return on equity don’t outperform meaningfully on their
tests. Other measures of return on equity did outperform sufficiently, however,
underlining the sensitivity of some factors to exactly how they are defined.
One lesson for investors is to be
careful about trying to make money by repeating what seems to have worked in the
past. If it was so easy, everyone would do it and it would stop working.
A former student of Mr. Fama,
Cliff Asness, founder of quantitative hedge-fund manager AQR Capital
Management, said he tries to avoid being caught out by false findings by
trading on anomalies he can explain, economically or through investor behavior.
To assess whether the market anomalies will continue, he looks for ones which
carried on after being identified, can be seen in other markets or asset
classes, and where minor changes to how they are defined don’t much affect the
result. These include most famously value, momentum and corporate quality,
among others.
Still, he worries that the
“awesome effort” in the new paper might lead some to overreact and reject all
factors, even those which Messrs. Hou, Xue and Zhang found evidence for.
“Many factors are demonstrably
silly, or are highly correlated versions of the same idea,” he said. “Where I
get worried is about overreaction [to the paper] and the cynicism it breeds.”
Investors are still likely to be
confused. There are well over 100 value and high-dividend ETFs in the U.S.
alone, tracking large, small or midsize stocks, based on different definitions
and often combined with other factors such as momentum, quality or low
volatility. Intelligently choosing between them would mean examining how
indexes are constructed and comparing to the long-term academic studies to see
which methodology was best; in practice for most investors there is little more
to go on than a few years of performance data and fees.
Worse still, the markets are
reasonably efficient. If it turns out that shares usually rise just after
Christmas or fall on Mondays when it rains in New York, traders will quickly
find a way to profit from the anomaly, and it will disappear.
The danger for investors who have
piled into “smart beta” ETFs betting on value or quality is that exactly this
happens. Small-capitalization companies stopped outperforming after the
landmark study identifying the so-called small-cap effect in 1981, for example,
and haven’t looked good since (see chart).
Any factor that might keep
working after discovery has to be hard to arbitrage away. For quality, a story
can be told of get-rich-quick investors overpaying for sexy high-growth
companies, but not—until recently—for shares of boring providers of steady
profits. Whatever the story, the more popular the factor becomes with
investors, the smaller its outperformance will be in future.
Messrs. Hou, Xue and Zhang
provide a handy dismissal of factors which didn’t even work that well in the
past. But ultimately no one knows whether even previously robust factors like
value and momentum will keep working.
Click
here for the original article from Wall
Street Journal.